Chapter 20 - Vectors
library(tidyverse)
20.3 - Important types of atomic vector
Problem 1
Describe the difference between is.finite(x)
and !is.infinite(x)
.
is.finite(x)
returns TRUE
for NaN
and NA
. !is.infinite(x)
returns FALSE
for NaN
and NA
. That’s because both functions test for finite/infinite and not missing. !
thus turns a FALSE
from a missing value into a TRUE
.
is.finite(NaN)
## [1] FALSE
!is.infinite(NaN)
## [1] TRUE
is.finite(NA)
## [1] FALSE
!is.infinite(NA)
## [1] TRUE
Problem 2
Read the source code for dplyr::near()
(Hint: to see the source code, drop the ()). How does it work?
It subtracts the first value from the second value and then sees if the difference is smaller than a tolerance of .Machine$double.eps^0.5
.
Problem 3
A logical vector can take 3 possible values. How many possible values can an integer vector take? How many possible values can a double take? Use google to do some research.
R has 2147483647 positive integers, 2147483647 negative integers, NA
, and 0L
. That sums up to 4.294967310^{9} possible values for integers. This numbers is ironically not an integer in R.
The maximum double on this computer is 1.797693110^{308} and the minimum double on this computer is 1.797693110^{308}. This machine floats to 53. Double can also take the values -Inf
, Inf
, NA
, and NaN
. Doubles can approximately take (.Machine$double.xmax * 2) * (10 ^ .Machine$double.digits) + 4
values. R can’t actually display this number.
Problem 4
Brainstorm at least four functions that allow you to convert a double to an integer. How do they differ? Be precise.
todo(aaron): I can think of ways to truncate decimals. I can’t think of ways to change the type of atomic vector.
2.2
## [1] 2.2
as_integer <- function(x) {
(x %/% 1)
}
typeof(as_integer(2.2))
## [1] "double"
as_integer <- function(x) {
x - x %% 1
}
as_integer(2.2)
## [1] 2
as_integer <- function(x) {
round(x)
}
as_integer(2.2)
## [1] 2
Problem 5
What functions from the readr package allow you to turn a string into logical, integer, and double vector?
parse_logical
, parse_integer
, and parse_number
20.4 - Using atomic vectors
Problem 1
What does mean(is.na(x))
tell you about a vector x? What about sum(!is.finite(x))
?
mean(is.na(x))
calculates the proportion of values in the vector that are missing.
sum(!is.finite(x))
calculates the number of values in the vector that are infinite, missing, or not a number.
Problem 2
Carefully read the documentation of is.vector(). What does it actually test for? Why does is.atomic() not agree with the definition of atomic vectors above?
is.vector()
tests if a vector is of the specified mode and has no attributes other than names.
is.atomic()
returns TRUE if the object is NULL
. In “R for Data Science”, NULL
isn’t considered a vector.
Problem 3
Compare and contrast setNames()
with purrr::set_names()
.
purrr::set_names()
is a snake case wrapper for setNames()
with tweaked defaults and stricter argument checking.
In particular, the argument x
is unnamed and and comes first and names are named and come second in purrr::set_names()
. Additionally, it tests to see if x
is a vector.
purrr::set_names()
is now the same as rlang::set_names()
so:
- If you do nothing, x will be named with itself.
- If x already has names, you can provide a function or formula to transform the existing names. In that case, … is passed to the function.
- If nm is NULL, the names are removed (if present).
- In all other cases, nm and … are coerced to character.
Problem 4
Create functions that take a vector as input and returns:
The last value. Should you use [ or [[?
Use [[
because it will always return only one value.
numbers <- c(1, 10, 93, 25, 43, NA, NA, 104, 10, NA, 25)
get_last <- function(x) {
x[[length(x)]]
}
get_last(numbers)
## [1] 25
The elements at even numbered positions.
get_evens <- function(x) {
index <- seq(from = 2, to = length(x), by = 2)
x[index]
}
get_evens(numbers)
## [1] 10 25 NA 104 NA
Every element except the last value.
get_all_but_last <- function(x) {
index <- 1:(length(numbers) - 1)
x[index]
}
get_all_but_last(numbers)
## [1] 1 10 93 25 43 NA NA 104 10 NA
Only even numbers (and no missing values).
get_evens <- function(x) {
index <- seq(from = 2, to = length(x), by = 2)
evens <- x[index]
evens[!is.na(evens)]
}
get_evens(numbers)
## [1] 10 25 104
Problem 5
Why is x[-which(x > 0)]
not the same as x[x <= 0]
?
x[-which(x > 0)]
returns NaN
and x[x <= 0]
does not return NaN
.
which()
returns integers of the positions that meet the criteria and aren’t NaN
. Adding -
to the index, drops the values meet the criteria and aren’t NaN
, but leaves the values that don’t meet the criteria or are NaN
.
Conditionals return NA
when tested against NaN
, so NaN
is dropped. This holds even if the opposite vector is used as an index. !
changes TRUE
to FALSE
and FALSE
to TRUE
but it leaves NA
as NA
.
x <- c(NA, -5, 10, 20, 0, NA, 10000, 10.1, NaN, Inf)
# 1
which(x > 0)
## [1] 3 4 7 8 10
#2
-which(x > 0)
## [1] -3 -4 -7 -8 -10
#3
x[-which(x > 0)]
## [1] NA -5 0 NA NaN
# 1
x <= 0
## [1] NA TRUE FALSE FALSE TRUE NA FALSE FALSE NA FALSE
# 2
x[x <= 0]
## [1] NA -5 0 NA NA
Problem 6
What happens when you subset with a positive integer that’s bigger than the length of the vector? What happens when you subset with a name that doesn’t exist?
The subset returns an NA
.
x <- c(1, 2, 3)
x[5]
## [1] NA
The subset returns an NA
with a name attribute of NA
.
x <- c(a = 1, b = 2, c = 3)
x["d"]
## <NA>
## NA
20.5 - Recursive vectors (lists)
Problem 1
Draw the following lists as nested sets:
list(a, b, list(c, d), list(e, f))
list(list(list(list(list(list(a))))))
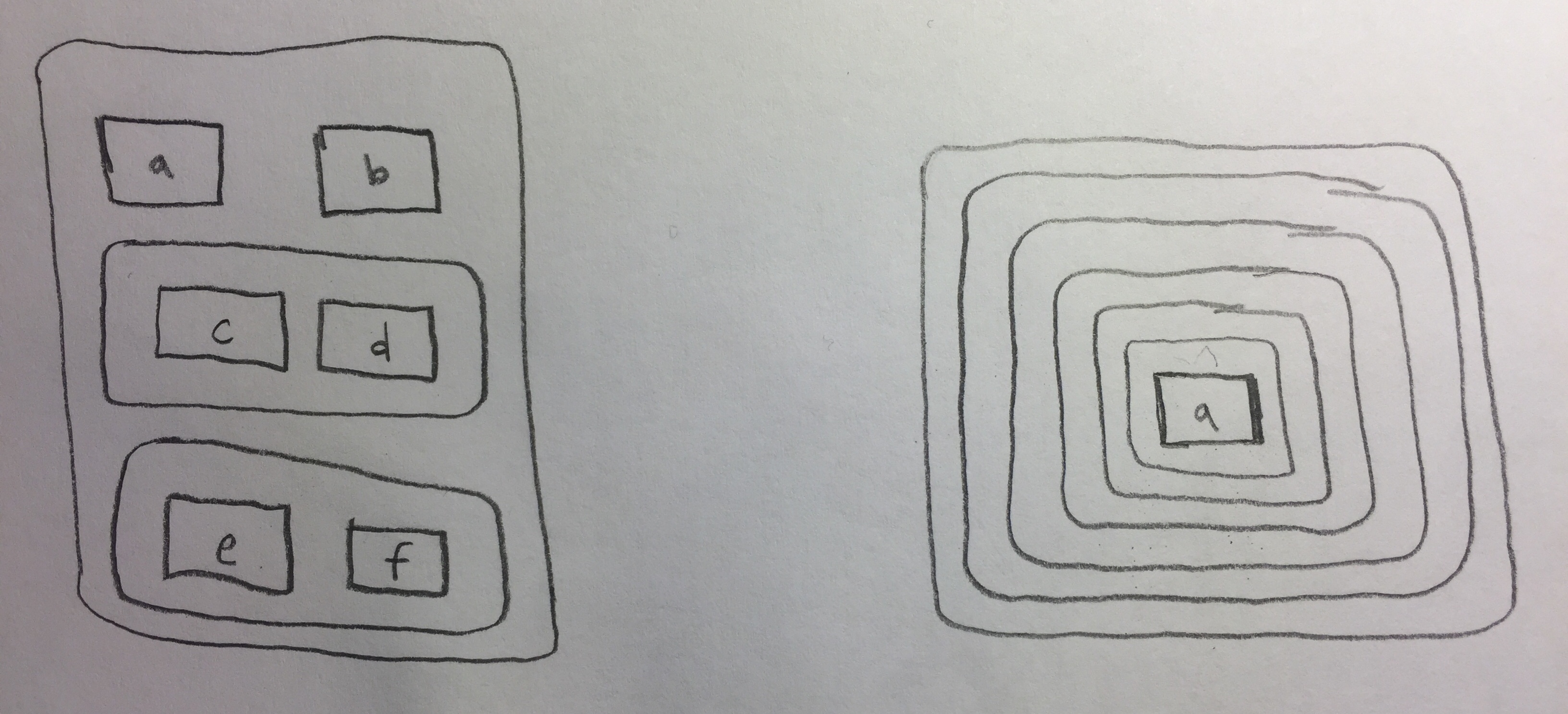
Problem 2
What happens if you subset a tibble as if you’re subsetting a list? What are the key differences between a list and a tibble?
[
returns a tibble
with the referenced columns. [[
returns a single vector with no attributes.
mtcars <- tbl_df(mtcars)
# tibble with just the first vector
mtcars[1]
## # A tibble: 32 x 1
## mpg
## <dbl>
## 1 21
## 2 21
## 3 22.8
## 4 21.4
## 5 18.7
## 6 18.1
## 7 14.3
## 8 24.4
## 9 22.8
## 10 19.2
## # ... with 22 more rows
# vector with no attributes
mtcars[[1]]
## [1] 21.0 21.0 22.8 21.4 18.7 18.1 14.3 24.4 22.8 19.2 17.8 16.4 17.3 15.2
## [15] 10.4 10.4 14.7 32.4 30.4 33.9 21.5 15.5 15.2 13.3 19.2 27.3 26.0 30.4
## [29] 15.8 19.7 15.0 21.4
A tibble
is a list of columns. The difference between a tibble and a list is that all of the elemtns of a data frame must be vectors of the same length while lists can have elements of different lengths.
20.7 - Augmented vectors
Problem 1
What does hms::hms(3600)
return? How does it print? What primitive type is the augmented vector built on top of? What attributes does it use?
It returns a vector with classes “hms” and “difftime”. The primitive type is double. It has attributes $units
and $class
.
Problem 2
Try and make a tibble that has columns with different lengths. What happens?
It returns an error: “Error: Column a
must be length 1 or 5, not 3”
tibble(a = 1:3,
b = 1:5)
Problem 3
Based on the definition above, is it ok to have a list as a column of a tibble?
Yes. columns are an important feature of tibbles.
tibble(a = list(c(1, 2, 3), c(1, 3), 1),
b = 1:3)
## # A tibble: 3 x 2
## a b
## <list> <int>
## 1 <dbl [3]> 1
## 2 <dbl [2]> 2
## 3 <dbl [1]> 3